Global X launched the first artificial intelligence ETFs in September 2016. Since then, it has returned 9.3% annualised versus 15.4% for the Nasdaq 100, a modest result in view of the hype that surrounds the sector.
Chart 1: Nasdaq 100 vs AI
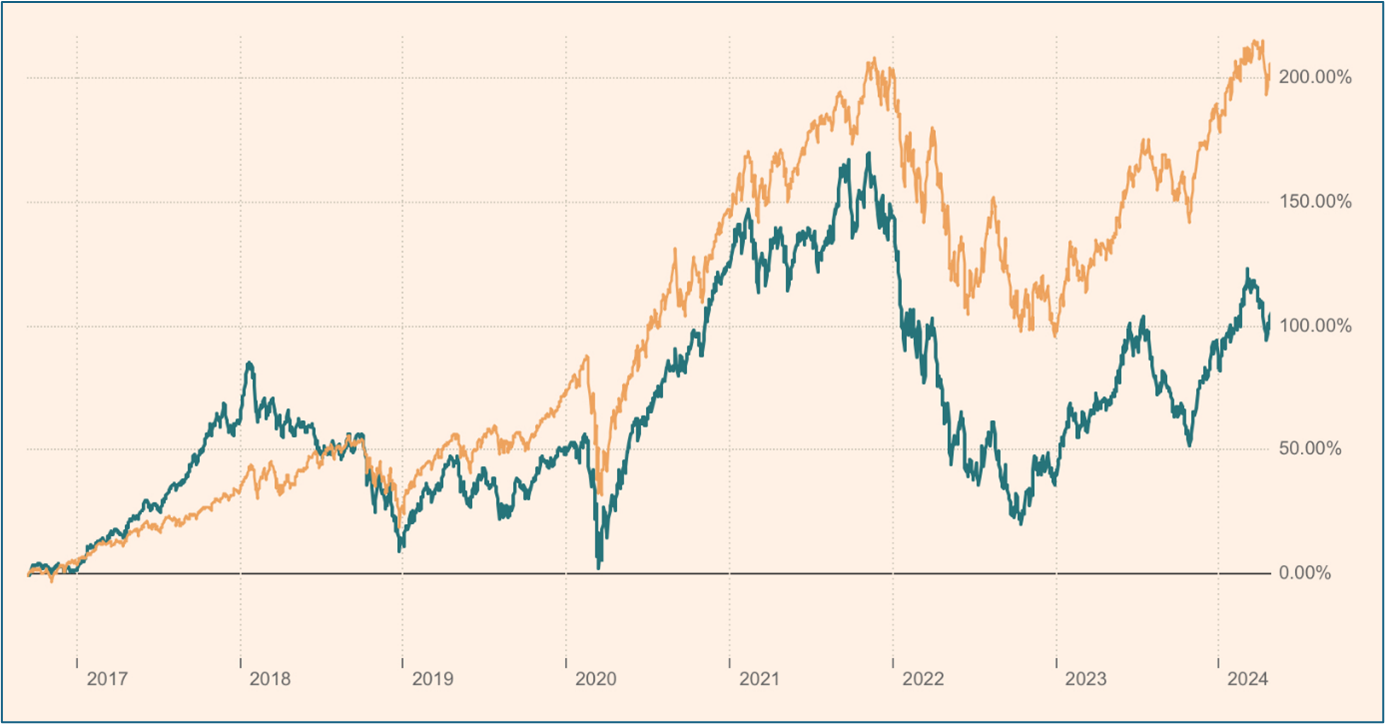
Source: Financial Times/Markets data. The orange line is the Nasdaq, and the blue line is the Global X
So, we are investing in AI, but, perhaps, we have not yet fully realised that we are increasingly investing (and living) with AI. As a result, an ETF buyer cannot avoid the question of whether, how and how much to use AI in his or her work. Above all, they must consider the ratio between the risks and the opportunities of using it. How much we know about AI is a thin slice of the uncharted waters that have yet to be explored.
In any case, giving a clear answer to this question is impossible. It all depends on where AI is used. We can only be enthusiastic about AI when it is used to perform boring, repetitive, or time-consuming tasks with little added value. Think of the Copilot platform for preparing PowerPoint presentations. What used to take hours of work and typographical skills that not everyone possesses now takes just a few minutes.
Its use in selecting information to support analysis and selection activities has been equally successful. Even the AI veteran, ChatGPT, is proving extremely useful in selectively finding ETFs with the characteristics to focus on. Things change, but for the worse, when the tasks to be performed become more intellectually demanding. Writing programming code (VBA Office, Python) you can also find some serious mistakes.
For example, ChatGPT once forgot to include the correlation matrix between asset classes when building a portfolio optimisation model. It is, however, a very obedient assistant. It never rejects error reports. In such cases, the AI assistant will apologise and re-execute the task until it achieves the expected results. Even when it comes to dealing with theoretical issues, it does sometimes make some severe mistakes.
In the words of Carlo Rubbia – physicist and winner of the Nobel Prize for Physics in 1984 – while "the PC is a fast idiot", AI is a fast intelligence because it learns from its mistakes.
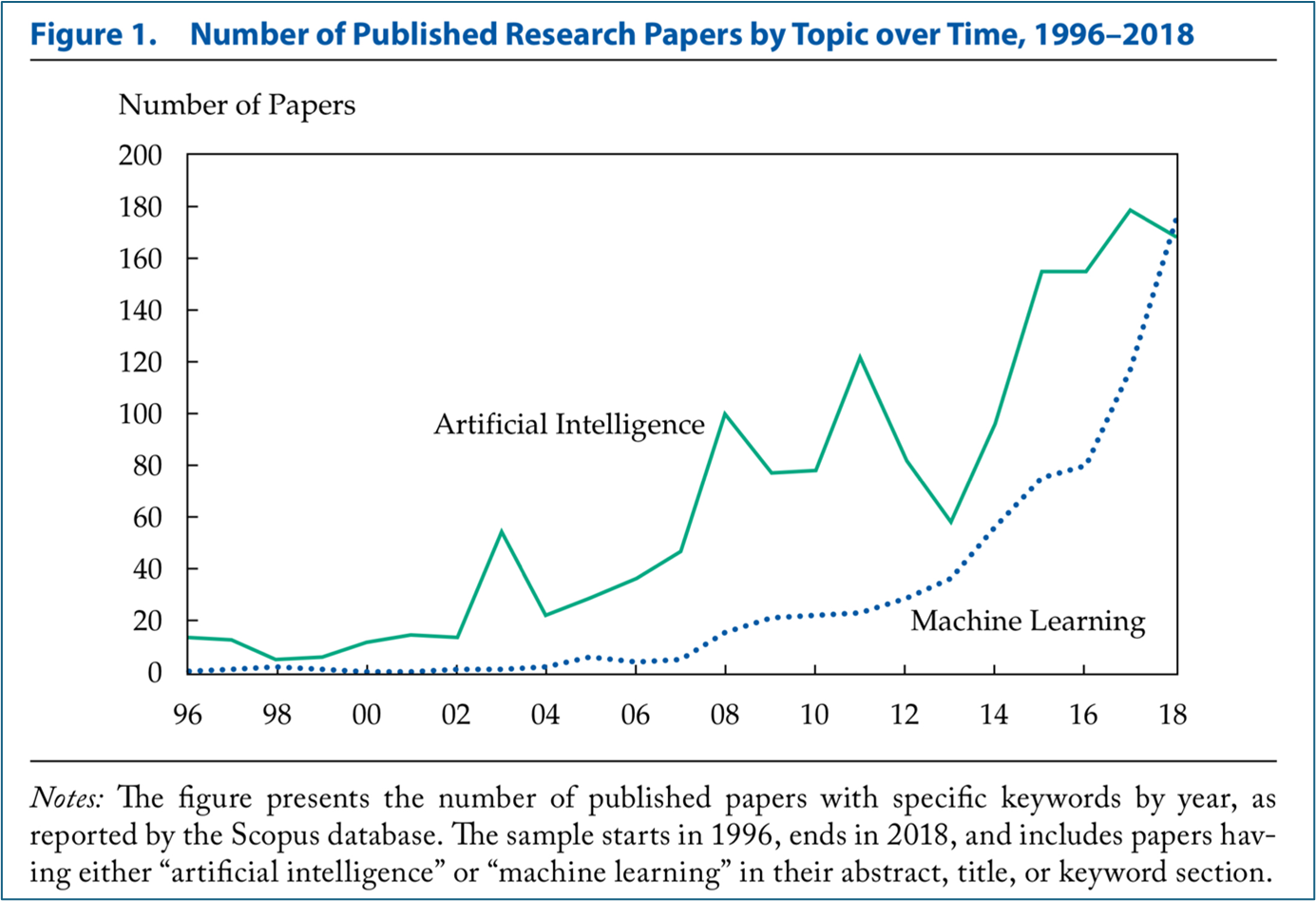
Source: Vv, Aa, Artificial Intelligence in Asset Management, CFA Institute research foundation/literature review,
However, all this is still folklore. Indeed, issues such as constructing asset allocations and estimating returns, volatilities and expected correlations are its most noble applications. AI is capable of data processing on a scale that would have been unthinkable just a short time ago. In the area of trading, it is generating operational signals like never before. It simplifies the analysis of the fundamental data of the companies that can be included in the portfolios in both the long and the short positions. It has been the catalyst for the development of robo-advisors, whose use is facilitated by the growth of ETFs in the markets. However, the decision-making process of an AI is not affected by biases and noise, as is the case with humans, on the contrary.
The real doubts and major concerns are about some of the limitations of today's AI, which could lead us to unintended consequences that are either serious or irreparable.
The potential risks – mostly hidden – that this new tool can introduce should be well understood by AI users. Risks that are related to the complexity and opacity of their operation. How would the AI algorithms react to a possible black swan event? And could they trigger a mechanism of cascading market crashes even in the absence of major adverse events? AI algorithms, in turn, can be fooled by the poor quality of the data they ingest and come to the wrong conclusions. Indeed, their weakness is that they always generate an answer even when there is none. In such a case, how much control would a human still have over such an algorithm?
There is no doubt that, after its long winter, the AI revolution has only just begun. Although its name dates to 1955, when John McCarthy held a workshop on artificial intelligence, it has a long way to go. The main risks are therefore related to the unintended consequences mentioned above.
The brand-new 737 MAX Lion Air JT610 on the flight from Jakarta on 29 October 2018, with 189 people on board, is a case in point. A few minutes after take-off, still climbing, the pilots reported the need to return due to technical problems with the flight control system. Unfortunately, there were no survivors when the plane broke up in the Java Sea a few minutes later. Why the tragedy? Even during the design and test phase, this aircraft had shown an excessive tendency to lift its nose when performing at maximum power compared to the older and more reliable 737. Boeing should have re-engineered the aerodynamics of the plane. Instead, it decided to solve the hardware issue with a software solution that was less demanding, less expensive, and less time-consuming. This is how the MCAS algorithm was born. It would independently modify the behaviour of the 737 MAX. The MCAS would intervene and make the appropriate corrections to eliminate the problem without the pilot having to intervene. This also saved time and money by eliminating the need for pilot training.
And so, a few poorly written lines of code have caused some accidents, killing hundreds, that traditional design techniques would almost certainly have prevented. Could financial algorithms, if they become widespread, trigger a market crash the likes of which we have never seen before?
To quote the playwright Fyodor Dostoevsky, “being smart is not enough to do smart things”. And this warning applies not only to AI. It also applies to those who use it carelessly in their line of work.
Edoardo Mezza is director and Filippo Arena is a private banker at Banca Patrimoni Sella & C.