Every investor has wished at some point, whether they admit to it or not, to have access to a crystal ball that could foresee the future. For all Tolkien fans out there, a palantír. For all Marvel fans, the Eye of Agamotto.
FactorResearch normally publishes work on factors because we can for the most part agree on their importance as drivers of excess returns, in large part due to the growing body of published, unpublished, and self-published work in this field of finance. Factors have been used to explain the past, and through somewhat heroic assumptions, to extrapolate into the future.
The problem is, of course, the latter use assumes the future will look like the past (which invites decades-old debates about how efficient markets are). This creates a catch-22 conundrum: either we assume that factors are good to forecast the future (which implies a strong intellectual hubris or we assume factors are not well equipped to help forecast the future (in which case we accept that making future investment decisions based on factors is not a good idea).
So, if traditional factors are not efficient nor useful to understand the forces that are driving disruption in public equities, what can we do?
Let’s break this problem in smaller parts. The first step towards uncovering the forces shaping change (disruptive or continuous) in public equities is to measure innovation.
Why focus on innovation?
In quantitative asset management, innovation has been until recently the Moby Dick of firm characteristics – that elusive item everyone knows is important, but no one could reliably model in a way that captured its desired properties – of which one is the well-established connection between innovation and future stock returns. It has been difficult to capture consistently (in a time series and cross-sectional form) firms’ exposure to innovation for several reasons:
The first place where investors look is Research and Development (R&D). This is a bad proxy because, among other reasons, it is a discretionary item that is treated as a short-term cost and is difficult to amortise.
There is a strong paradox when it comes to a firm’s decision to disclose the details of their R&D: on one hand, companies want to let investors know about it but on the other hand, they don’t want to give up a competitive advantage by disclosing too much.
Sell-side analysts face career concerns that make them averse to making calls based on innovation given the uncertain long-term nature and low hit rate of these calls
Public equity markets provide a very poor backdrop to measure innovation given the agency issues associated with the financing of innovative ideas and concepts.
What to do then? One solution is to look at alternative markets where successful innovation is identified and understand how it is rewarded. The challenge is that we need more tools than we have traditionally used in factor research. Specifically, we need to use natural language processing.
Introduction to natural language processing
The use of applications constructed via natural language processing (NLP) is present in almost every aspect of our lives, from Google searches to Netflix recommendations. These techniques, when applied to all publicly available information on alternative markets allows us to create a model of innovation based on the support (financing), attention (news), and protection (patent activity) surrounding funding events in the non-public space. The result is the identification of the innovative concepts embedded in swaths of unstructured data.
The chart below shows a very small (emphasis on small) section of the data, where the cream and yellow-colored diamonds represent concepts like “cryptocurrency” or “hydrogen fuel”, the circles represent conventional industries, like “banks” or “diversified metals” and the gray lines represent the connectivity between concepts and industries. For instance, notice how “bitcoin” is connected to “gold” – readers that are into crypto will immediately recognise this as the disruptive threat that bitcoin represents to gold as a store of value.
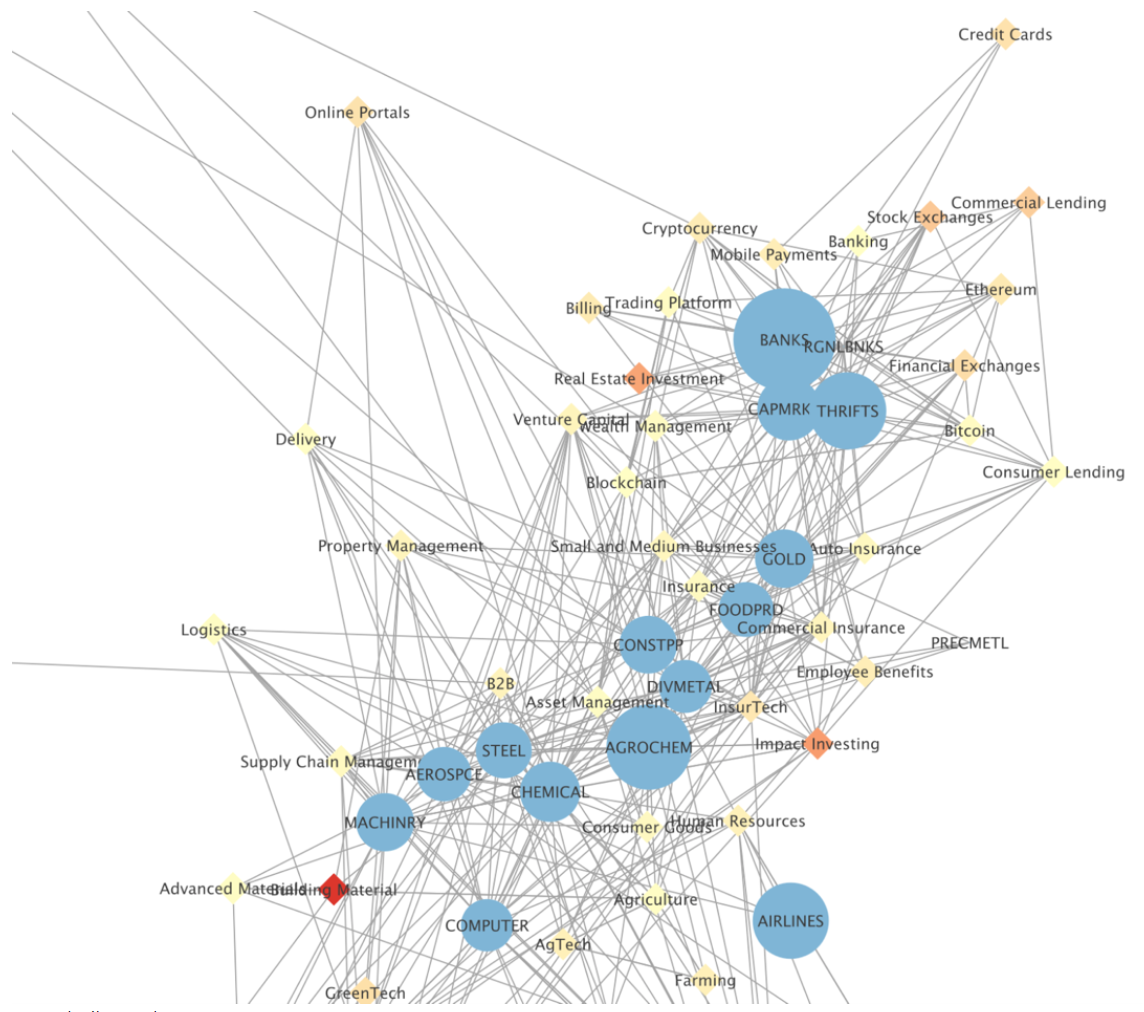
Source: Pluribus Labs
Identifying forces of disruption
More broadly, our analysis of innovation indicates that in the medium term some industries likely to experience disruptive innovation are precious metals, regional banks, airlines, agrochemical, and utilities. It is important to remember that disruption is a process, not a single event.
Because disruption takes time, incumbents frequently overlook potential disruptors and their transformational impact. Most importantly, some disruptive innovations succeed, others don’t. With that in mind, let’s look at the top concepts likely to disrupt two industries: consumer financial services and metals and mining.
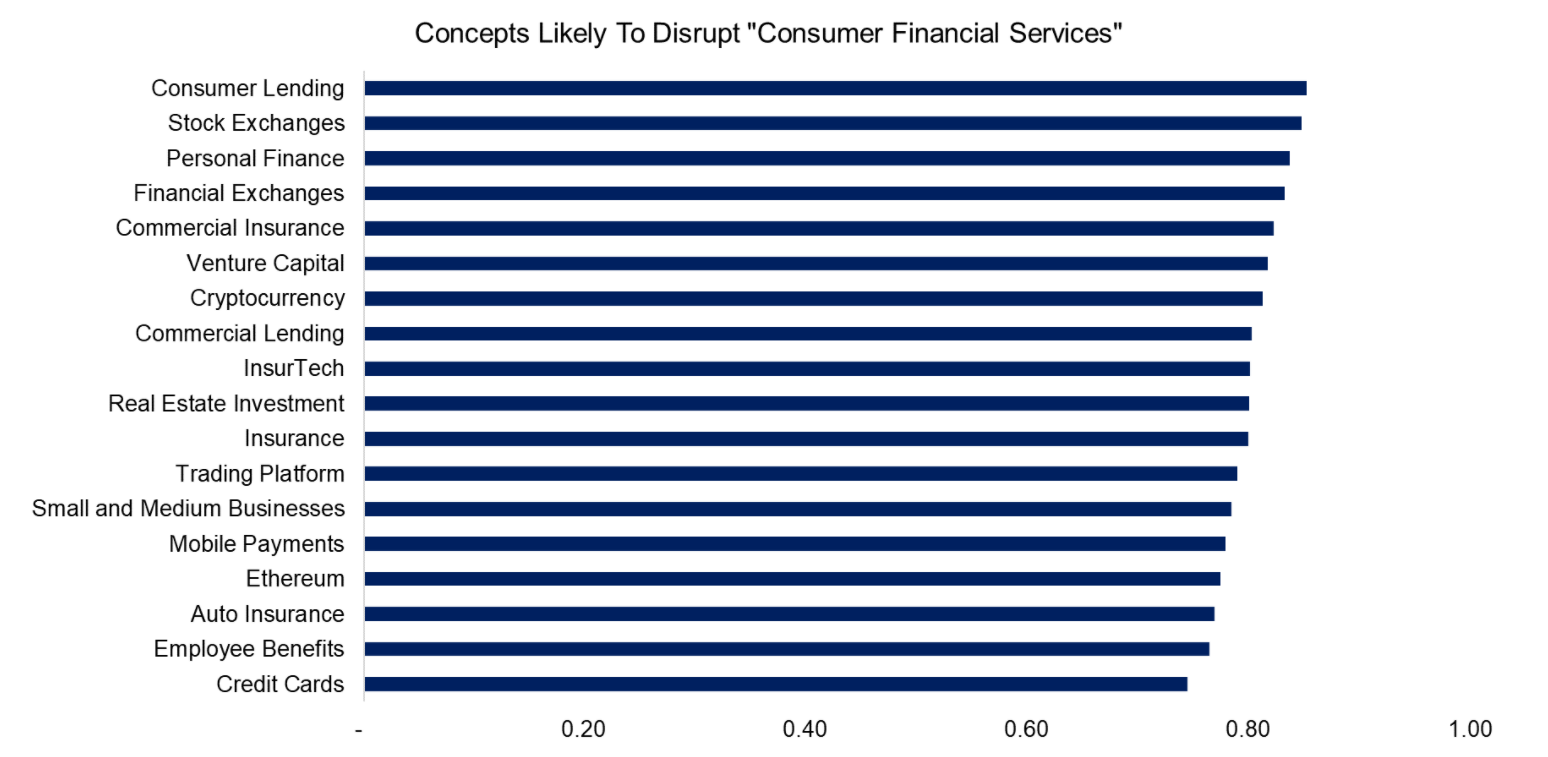
Source: Pluribus Labs
The most important disruptive trend in consumer financial services is the one represented by the nascent adoption of DeFi (decentralised finance) technologies. Growth is already steep and could still accelerate in the near term. Sell-side institutions have reported that DeFi has generated $2trn in total investment interest, as of April 2021, and that this figure has doubled in the first third of 2021 alone.
In an environment where political tensions run high and economic uncertainty abounds, there is obvious appeal to a technology that is global, permissionless, flexible, transparent, and interoperable. Some of the most promising DeFi projects are native lending tokens, which allow lenders to passively farm income while borrowers get access to attractively priced capital to use in numerous traditional capacities.
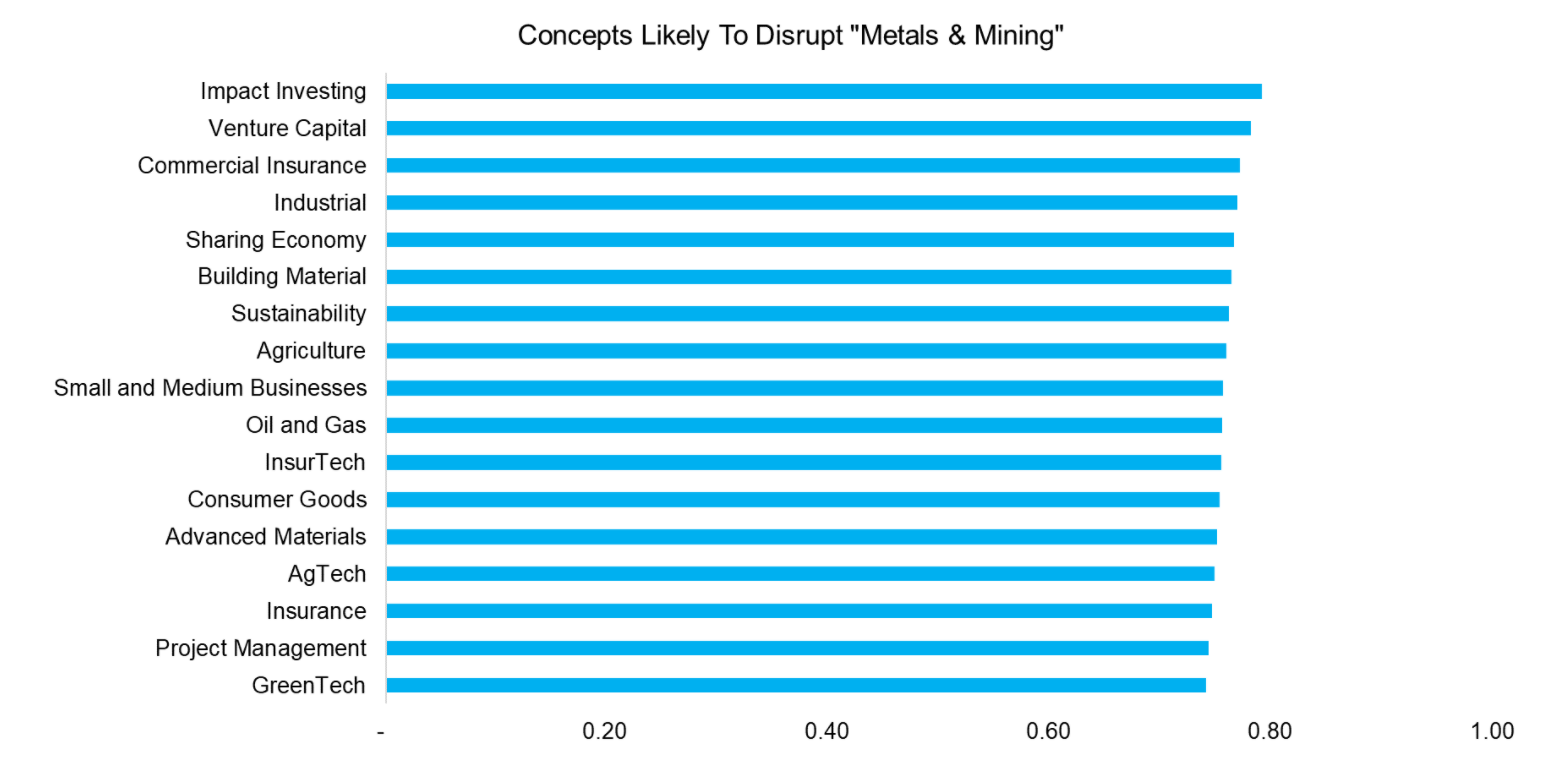
Source: Pluribus Labs
Looking at metals and mining, it is interesting that the two most disruptive concepts are Impact Investing and Venture Capital. Within metals, steel is one of the most integral components of modern civilisation, serving as the skeleton for buildings, roads, railways, and other components of contemporary infrastructure.
At surface, many would assume that there is little technological innovation impacting steel production. However, “smart” plants and other environmentally friendly innovations are displacing and replacing traditional production environments with highly automated, digitalisation-enabled facilities which unlock economic and ecological efficiencies throughout the production process.
Climate-driven innovation is directly aiming at steel production given it is a massive source of pollution, generating 7% to 9% of all direct emissions from fossil fuels globally. We should expect to hear more about projects aiming to create fossil-free processes in the steel industry soon.
Technological innovation is also enhancing the viability of recycling in the industry. Steel production generates high volumes of waste materials like dust, fines, and mill scale. Innovations in by-product recycling are allowing proactive producers to convert these residual materials into useful and profitable resources.
Conclusion
These are just glimpses of the power and potential of natural language processing when used to answer a well-defined question. Potential extensions and applications of this methodology create exciting opportunities to asset managers and allocators alike.
Wachi Bandara is CIO and Rodolfo Martell is head of portfolio strategy at Pluribus Labs